Diff-Foley: Synchronized Video-to-Audio Synthesis with Latent Diffusion Models
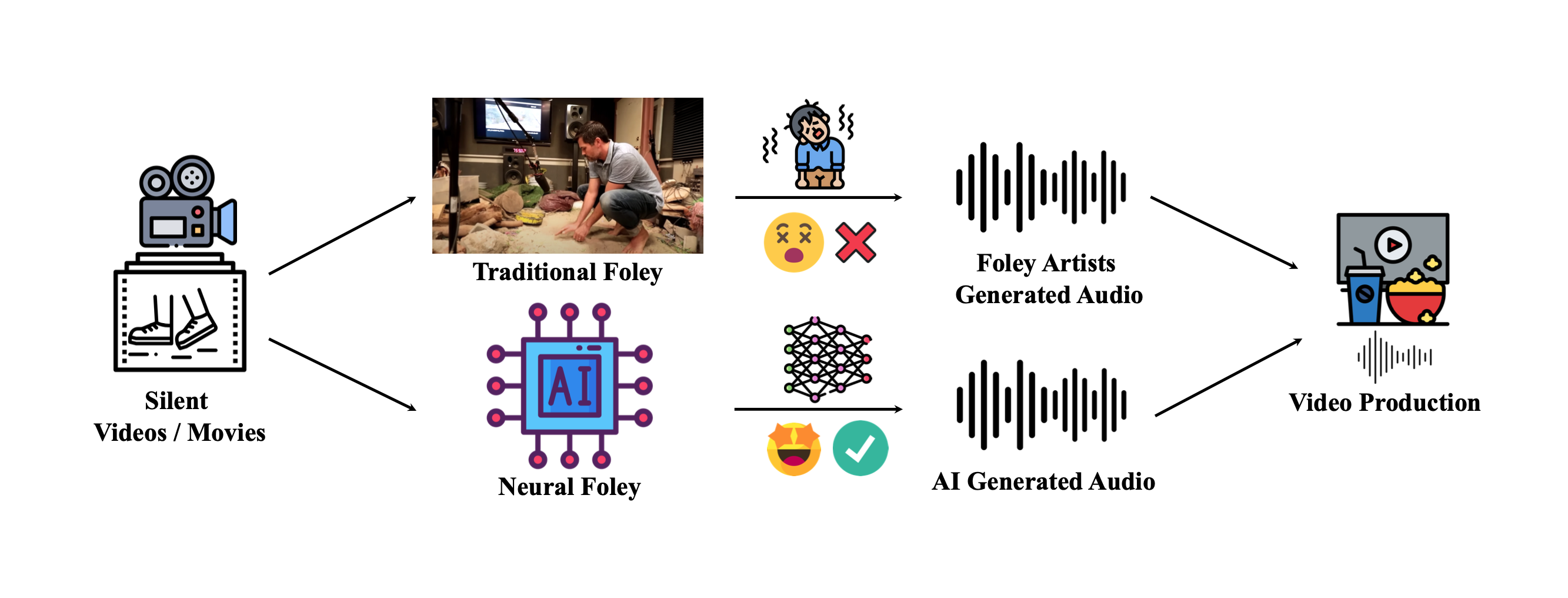
(Note: It is recommended to use Chrome browser for optimal content display.)
Abstract
The Video-to-Audio (V2A) model has recently gained attention for its practical application in generating audio directly from silent videos, particularly in video/film production. However, previous methods in V2A have limited generation quality in terms of temporal synchronization and audio-visual relevance. We present Diff-Foley, a synchronized Video-to-Audio synthesis method with a latent diffusion model (LDM) that generates high-quality audio with improved synchronization and audio-visual relevance. We adopt contrastive audio-visual pretraining (CAVP) to learn more temporally and semantically aligned features, then train an LDM with CAVP-aligned visual features on spectrogram latent space. The CAVP-aligned features enable LDM to capture the subtler audio-visual correlation via a cross-attention module. We further significantly improve sample quality with double guidance . Diff-Foley achieves state-of-the-art V2A performance on current large scale V2A dataset. Furthermore, we demonstrate Diff-Foley practical applicability and generalization capabilities via downstream finetuning.
Evaluation Results
For evaluation, we compared our results with SpecVQGAN and Im2Wav on VGGSound dataset with the following metrics: FID, KL, IS. Also, we introduced another metric - Alignment Accuracy (Align Acc) to evaluate the audio synchronization and audio-visual relevance. We also show the models average inference time on generating a batch of 64 audio samples. The results are presented in the table below.
Model | IS ↑ | FID ↓ | KL ↓ | Align Acc ↑ | Infer. Time ↓ |
---|---|---|---|---|---|
SpecVQGAN (ResNet50) | 30.01 | 8.93 | 6.93 | 52.94 | 5.47s |
Im2Wav | 39.30 | 11.44 | 5.20 | 67.40 | 6.41s |
Diff-Foley , Classifier Free Guidance (Ours) | 53.34 | 11.22 | 6.36 | 92.67 | 0.38s |
Diff-Foley , Double Guidance (Ours) | 62.37 | 9.87 | 6.43 | 94.05 | 0.38s |
Demos
It is recommended to use earphones to hear the demos videos, raise the volume and zoom in the videos.
Note: We only demonstrate the SpecVQGAN baselines here. For Im2Wav, the results are similar to SpecVQGAN.
Ground Truth
Baseline
Sample1 (Ours)
Sample2 (Ours)
Sample3 (Ours)
Playing Drum
Slamming Glass
Playing Golf
Billiards
Diving
Gun Shooting
Concert
Underwater Bubbling
Bouncing On Trampoline
Pidgeons Cooing
Car Start Moving
Playing Drum
Playing Bowling Ball
Chopping Wood
Playing Tennis
Downstream Finetuning
We demonstrate the downstream finetuning results of Diff-Foley on EPIC-Kitchens datasets in the following videos.
Ground Truth
Sample1 (Ours)
Sample2 (Ours)
Sample3 (Ours)
BibTeX
If you find our work intriguing, inspiring or useful to your research, please consider citing:
@misc{luo2023difffoley,
title={Diff-Foley: Synchronized Video-to-Audio Synthesis with Latent Diffusion Models},
author={Simian Luo and Chuanhao Yan and Chenxu Hu and Hang Zhao},
year={2023},
eprint={2306.17203},
archivePrefix={arXiv},
primaryClass={cs.SD}
}